Link to Talk Video: https://mediaspace.illinois.edu/media/t/1_oyj2ojaq
Abstract: The success of supervised machine learning in recent years crucially hinges on the availability of large-scale labeled data, which is often time-consuming and expensive to collect. In light of this challenge, there is a surge of recent interest in learning the so-called invariant representations, and it has found abundant applications in domain adaptation / generalization, fairness, privacy-preservation learning, multilingual representation learning, etc. However, it is not clear what price we have to pay in terms of task utility for such universal representations. In this talk, I will discuss my recent work on understanding and learning invariant representations, with a special focus on its application in domain generalization.
In the first part, I will focus on understanding the costs of existing invariant representations by characterizing a fundamental tradeoff between invariance and utility. In particular, I will use domain adaptation as an example to both theoretically and empirically show such tradeoff in achieving small joint generalization error. This result also implies an inherent tradeoff between invariance and utility in both classification and regression settings, and I will introduce an information-plane analysis to characterize the Pareto frontier between them. In the second part of the talk, I will focus on designing learning algorithms to escape the existing tradeoff and to utilize the benefits of invariant representations. Finally, I will outline several potential future research directions on learning with invariant representations.
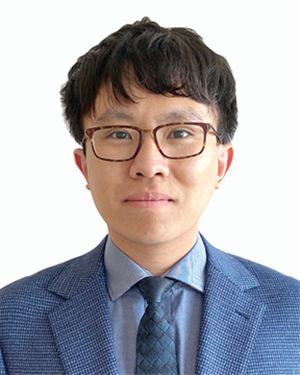
Bio: Han Zhao is a tenure-track Assistant Professor in the Department of Computer Science at the University of Illinois at Urbana-Champaign (UIUC). His research interests are broadly in machine learning, with a focus on representation learning and probabilistic circuits. His long-term goal is to build a unified framework that provides common semantics for learning and reasoning, by developing invariant representations that generalize across different environments as well as efficient inference engine that allows exact and tractable probabilistic reasoning. Before joining UIUC, he was a machine learning researcher at D.E. Shaw. He obtained his BEng degree in Computer Science from Tsinghua University, MMath degree in mathematics from the University of Waterloo, and his Ph.D. degree in Machine Learning from Carnegie Mellon University.
Password: csillinois
Part of the Illinois Computer Science Speakers Series. Faculty Host: Heng Ji